

2025 10th International Conference on
Biomedical Signal and Image Processing
Important Dates
- Submission Deadline: Before Jul. 05, 2025
- Notification of Acceptance: Jul. 20, 2025
- Registration Deadline: Before Jul. 25, 2025
- Conference Date: August 1-3, 2025
Authors can attend the conference with paper publication or without publication. For paper publication, full paper should be submitted. For presentation only, abstract should be submitted.
Contact Us
icbip@cbees.net
+852-3500-0799 (English)
-
+86-28-86528465 (Chinese)
-
Ms. Jamila Liu
-
Monday-Friday, 9:30-18:00
-
Co-Sponsored By
-
-
Patrons
-
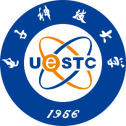
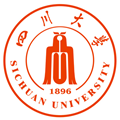
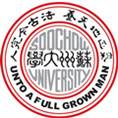
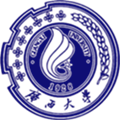
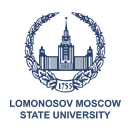
Prof. Donghyun Kim(SPIE Fellow)
Yonsei University
Donghyun Kim received B.S. and M.S. from Seoul National University in Electronics Engineering. He graduated from the Massachusetts Institute of Technology with Ph.D. in electrical engineering in the area of novel multi-dimensional display technologies and smart optical filters. He worked on next generation fiber-optic access communication systems at Corning Inc. as a senior research scientist and then investigated cellular biophotonic sensors for cell-based assays at Cornell University as a postdoctoral fellow. He has been leading Biophotonics Engineering Laboratory of Yonsei University, Seoul, Korea. The main theme of his research at Yonsei has been focused on sensing and imaging applications in biomedical engineering based on near-field manipulation by plasmonic techniques and metastructures. He has given 100+ invited lectures on related topics and written more than 200+ peer-reviewed journal and conference publications on nano and biophotonics, many of which were the results of domestic and international collaboration. He also holds 30+ international patents. Dr. Kim served as Undergraduate Chair of the School of Electrical Engineering of Yonsei University and chaired international conferences including CLEO Pacific Rim 2024. He is currently Vice President of the Optical Society of Korea. He is a Fellow of the SPIE and a Senior Member of the IEEE.
Prof. Haiteng Jiang
Zhejiang University
Jiang Haiteng is
a National High-Level Young Talent, Chief Young
Scientist of the China Brain Project, and Director
of the Clinical Base Office of the National Key
Laboratory of Brain-Machine Intelligence. He is a PI
at MOE Frontier Science Center for Brain Science and
Brain-Machine Integration, the Affiliated Mental
Health Center of Zhejiang University School of
Medicine and Liangzhu Laboratory. His team has been
dedicated to developing novel brain information
analysis methods, exploring cognitive and brain
disease mechanisms, and facilitating clinical
translation. He currently leads three national-level
projects and his representative research findings
have been published in high-impact journals such as
Advanced Science, Annals of Neurology, and
Neuropsychopharmacology as a corresponding or first
author. He has received several awards, including
the LiZao Neuroscience Award from Zhejiang
University School of Medicine, the Emerging
Countries Travel Scholar Award at the 21st
International Conference on Biomagnetism, and the
2018 Neuroscience Society Hot Topic Award.
Speech Tit;le: "Translational Psychiatry with
Real-World Clinical EEG"
Abstract: Real-world data (RWD) research involves
the analysis of practical, heterogeneous data
collected during routine clinical practice. Unlike
controlled trial data, RWD is characterized by
diversity, non-structural formats, complexity, and
imperfections, posing challenges but also offering
valuable insights into real clinical scenarios.
Electroencephalography (EEG), a non-invasive
technique for monitoring brain electrical activity,
has become a critical tool in diagnosing and
researching neurological and psychiatric disorders
such as epilepsy, depression, and sleep disorders.
This report highlights our research group’s progress
in leveraging deep learning (DL) and transfer
learning (TL) techniques to automate sleep staging
and enhance the precision of psychiatric disorder
diagnoses using real-world EEG and polysomnography
(PSG) data. Sleep staging, traditionally a
labor-intensive manual process, benefits from DL’s
ability to extract complex patterns from raw EEG
signals, improving accuracy and efficiency.
Meanwhile, TL enables the adaptation of pre-trained
models to diverse patient populations, addressing
data scarcity and variability in clinical settings.
By integrating real-world EEG and PSG data, our work
aims to bridge the gap between research and clinical
application, ultimately advancing personalized
medicine and improving diagnostic outcomes for sleep
and psychiatric disorders.
Prof. Dakun Lai
University of Electronic Science and Technology of China (UESTC)
Professor Lai is
currently the director of the Biomedical Imaging and
Electrophysiology Lab at the University of
Electronic Science and Technology of China (UESTC),
and the Vice Chairman of Chinese Heart Rhythm
Society (CHRS). He received his Ph.D. in Medical
Electronics from Fudan University in 2008. Then he
completed a three-year Postdoctoral Associate in
Biomedical Engineering at the University of
Minnesota, USA. From 2012, he has been on the
faculty of the School of Electronic Science and
Technology, UESTC, China, where he was appointed as
a Professor of Electrical Science and Technology.
Dr. Lai is members of IEEE and the Engineering in
Medicine and Biology Society, and the member of
American Heart Associate. He is also an editor and
reviewer of several international journals. He has
published 80 peer-reviewed papers in Circulation,
Physics in Medicine and Biology, IEEE TITB, IEEE
Sensors J etc. and holds 30 Chinese Patents. His
research interests and main contributions include
medical electronics, bioelectromagnetism and
cardiac/nural electrophysiology. He has pioneered
the development of various wearable ECG devices with
flexible dry electrodes and made significant
contributions to deep learning based bioelectrical
signal analysis, and detection and prediction of
severe cardiac arrhythmias and neuro-disorder.
Dr. Fan Lin
Tongji Hospital, Tongji
Medical College, Huazhong University of Science and
Technology
Lin Fan has been working at Tongji
Hospital, Tongji Medical College, Huazhong University of
Science and Technology, since his graduation in 2011. He is
a member of the Chinese Non-invasive Electrophysiology
Professional Committee and the Electrocardiography Branch of
the Wuhan Medical Doctor Association. Lin specializes in the
diagnosis of various arrhythmias, pacemaker programming and
analysis, as well as esophageal and intracardiac
electrophysiological examinations. He was awarded the 3rd
"China Non-invasive Cardiac Electrophysiology" Promotion and
Application Award. Currently, his research focuses on
artificial intelligence in cardiovascular physiological
signals. He leads one National Natural Science Foundation of
China (NSFC) General Program and one Tongji Hospital Youth
AI Project. His key findings have been published in renowned
journals, including Med (2 papers), European Heart
Journal-Digital Health, IEEE Journal of Biomedical and
Health Informatics (JBHI), and the Chinese Journal of
Cardiac Arrhythmias. Additionally, he holds multiple
authorized invention patents.
Speech Tit;le: "AI in Atrial Fibrillation
Research: Clinician Perspectives on Challenges and
Opportunities"
Abstract: This clinician-focused analysis explores
AI's integration in atrial fibrillation (AF)
research, revealing significant opportunities
alongside challenges. AI demonstrates high
specificity in detecting paroxysmal AF episodes (≥6
minutes) and distinguishing AF from multiple other
arrhythmias using heart rhythm data. Critically,
clinician-AI collaboration achieves 100% diagnostic
accuracy while reducing physician workload by 76.7%,
optimizing clinical efficiency. Furthermore, the
HBBI-AI model predicts AF risk from sinus rhythm
intervals alone, outperforming traditional models
(e.g., C2HEST), with explainability analysis linking
risk to autonomic imbalance—suggesting pathways for
personalized prevention. While these advances signal
significant opportunities in real-time monitoring
and precision risk assessment, clinicians emphasize
persistent challenges, including model
interpretability, integration into existing
workflows, and validation across diverse
populations. This clinician-centered perspective
underscores AI’s dual role as both a transformative
tool and an evolving solution requiring
collaborative refinement to realize its full
potential in AF research and care.
Assoc. Prof. Liu
Yan
Sichuan University
Liu Yan, Associate Professor. She has
been engaged in medical image analysis, pattern recognition,
and machine learning related work. Her specific research
directions includes image segmentation, image registration,
deep learning, etc. At present, She have published multiple
high-quality papers in authoritative academic journals and
conferences, and have been invited multiple times to give
presentations at mainstream conferences. She has been the PI
of multiple national and provincial-level projects,
including the National Natural Science Foundation of China
and key research and development projects in Sichuan
Province.
Speech Tit;le: "AI in Atrial Fibrillation
Research: Clinician Perspectives on Challenges and
Opportunities"
Abstract: This report focuses on geometric shape
feature-guided medical image segmentation. It
explores how to leverage anatomical characteristics
to enhance segmentation accuracy. Relevant methods
integrating shape constraints into segmentation
models are elaborated, with experiments verifying
their effectiveness in improving boundary precision
and reducing false segmentation in medical images.
Dr. Rajeev K. Singla West China Hospital, Sichuan University,
China
Dr. Rajeev Kumar (also known as
Rajeev K. Singla) is an assistant researcher at the
Institutes for Systems Genetics, West China Hospital,
Sichuan University. He has extensive experience in the
fields of natural products, metabolic disorders,
neurological diseases, infectious diseases,
chemoinformatics, and public health. He is the founder and
editor-in-chief of Indo Global Journal of Pharmaceutical
Sciences, associate editor of Journal of Translational
Medicine, Journal of Alzheimer’s Disease, Frontiers
in Pharmacology, and Frontiers in Nutrition, a member of the
Topical Advisory Panel of Cancers (MDPI), a member of the
Advisory Board of Heliyon (Cell Press), and a member of the
Editorial Board of BMC Complementary Medicine and Therapies
and CNS & Neurological Disorders - Drug Targets. He is among
the top 2% of scientists according to Stanford University,
Scopus, and Elsevier reports (based on publications in 2022,
2023, and 2024). He has published more than 140 papers.
According to Scopus, he has published papers with 670
co-authors from 40 countries so far. According to Web of
Science, he has 447 peer review records and 298 verified
editing records. To date, he has published 4 books and 19
chapters. He serves as an expert reviewer for the Sichuan
University Innovation Training Program Academy and
participates in guiding the College Students Innovation and
Entrepreneurship Competition. In addition, he was awarded
the 2024 Sichuan Province Foreign High-level Talent
Certificate this year and won the second prize for the
2022-2023 Suzhou Natural Science Excellent Academic Paper
Award (SZLW2024084). In 2024, he successfully applied for a
Sichuan Provincial Science and Technology Department project
to find alternative therapies extracted from coconut shells
to fight Pseudomonas aeruginosa (Project No.: 2025YFHZ0213).
Assoc. Prof. Zhiwei Wang
Huazhong University of Science and Technology
Assoc. Prof. Gaoyan
Zhang
Tianjin University,
China
Copyright (c) 2025 www.icbip.org. All rights reserved. ICBIP 2025.